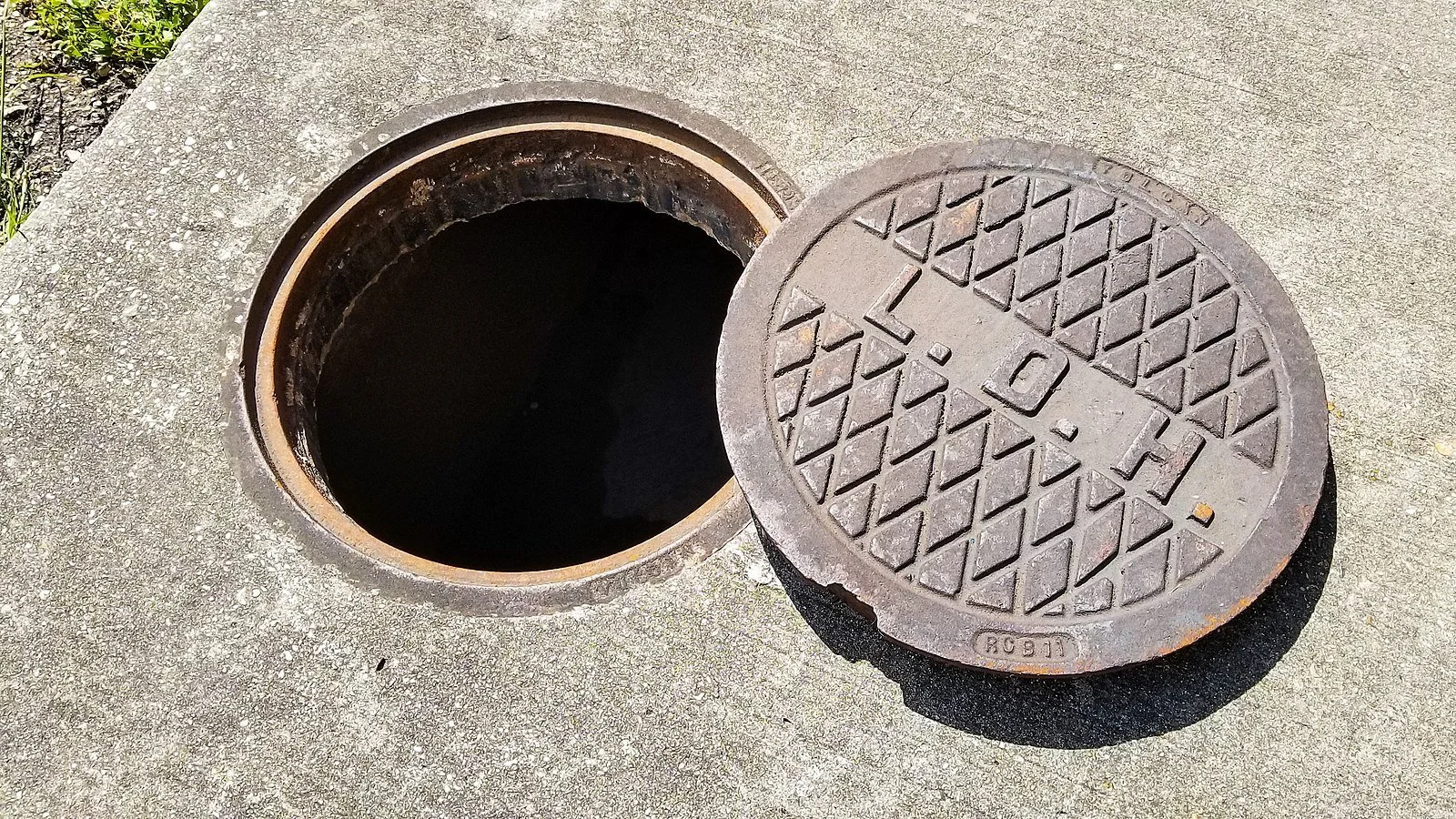
Four years ago we set out to answer a question: why do some developers get incredibly high paying jobs with multiple offers while others seem to struggle on the job search, regardless of their technical differences?
Landing a job as a software engineer in the Bay Area is a difficult and daunting process. Keeping up with relevant skills can be overwhelming, even when you’re a veteran…
On December 1st, 2018 Pariss Chandler, a front end web developer, made a call on Twitter that sparked a movement. She asked what may seem like a simple question yet it had a huge impact…
The House Robber Problem is a popular question in software engineering interviews, but not because of it’s practical applications. We’ve seen a lot of competent engineers struggle with this problem, because, perhaps unsurprisingly, the optimal approach uses dynamic programming…
As an engineer when you walk into the interviewing room, you never know who you are going to meet or what questions they are going to ask you…
There you stand, alone staring at a whiteboard with the hiring manager standing behind you. They ask you to solve a problem you’ve never seen before. Have you ever had the experience of freezing after being given a particularly hard problem?
Working at Outco for the better part of a year now, I’ve noticed some patterns in how engineers learn the material and what kinds of roadblocks they run into…